(通讯员:李家艺)受土耳其工业技术部的委托,武汉大学遥感信息工程学院黄昕教授团队将遥感和深度学习应用于2023年2月6日土耳其地震的倒塌房屋检测。在受地震影响较大的六个城市(Islahiye, Nurdagi, Adiyaman, Kahramanmaras, Turkoglu, Antakya)中,共检测出了2377栋由于地震而倒塌的房屋,并提供了所有倒塌房屋的中心坐标。土耳其工业技术部丝路发展局(Silkroad Development Agency)秘书长Burhan AKYILMAZ专门致信表示感谢:“该团队及时、有效、精确地检测了倒塌房屋信息,包括数量与位置(Their techniques are timely, effective and accurate for detecting the information of the collapsed buildings, e.g., number and location...,their contributions are highly appreciated)”。
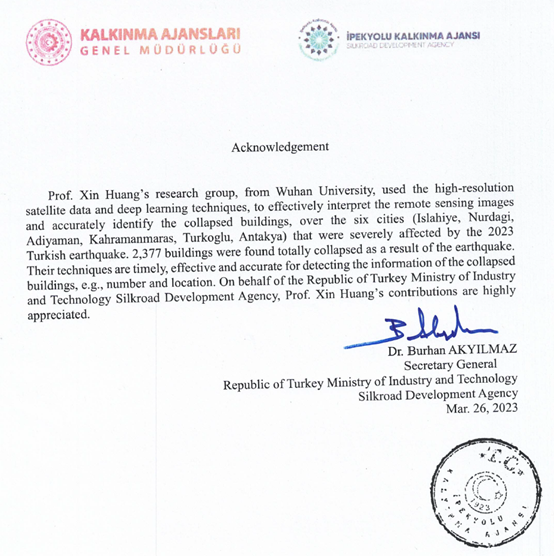
土耳其工业技术部丝路发展局(Silkroad Development Agency)感谢信
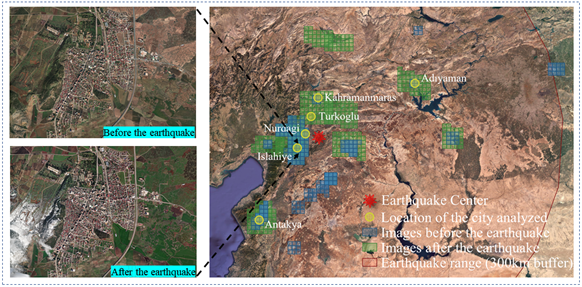
图1右侧图片展示了与地震相关的数据信息。其中红色点表示地震中心;黄色点表示受地震影响最严重的六个城市;绿色方框表示震后可用的影像;蓝色方框表示震前可用的影像
黄昕团队此次检测采用了Maxar高分辨率遥感影像(分辨率0.5米),将影像和少量的人工标注送入开源数据集预训练的语义分割网络,网络的输出即为坍塌建筑的预测。该检测手段突破了两个技术难题:(1)通过半监督学习,增加了可靠样本,解决了样本标注少、耗时费力等问题;(2)语义分割的类别不平衡问题,即:倒塌房屋在应用场景中属于稀少类别,从而导致模型过拟合、泛化不足、虚警高等问题,从而将网络预测结果和OSM叠置,获得坍塌前的建筑数量及位置。
在6个城市中共检出2377栋坍塌(不包括轻微受损)的建筑。下图分别展示了Islahiye、Nurdagi、Adiyaman、Kahramanmaras、Turkoglu、Antakya的检测结果。其中左列为震前影像,中列为震后影像与倒塌建筑的预测的叠加图,右列为震后影像与震前建筑足迹的叠加图。

图2.倒塌房屋检测示例(Islahiye)

图3.倒塌房屋检测示例(Adiyaman)
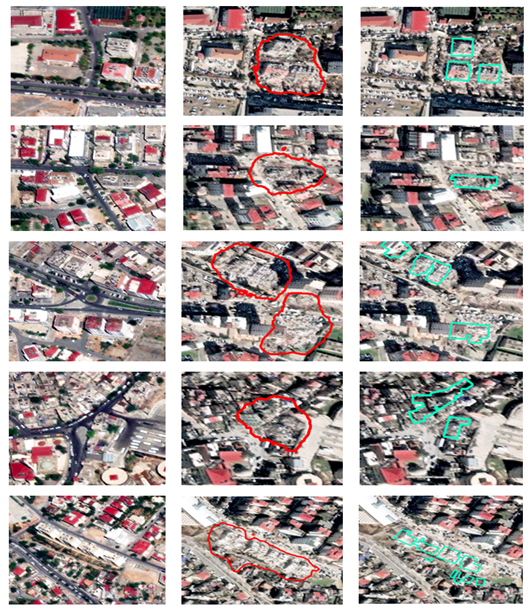
图4.倒塌房屋检测示例(Kahramanmaras)
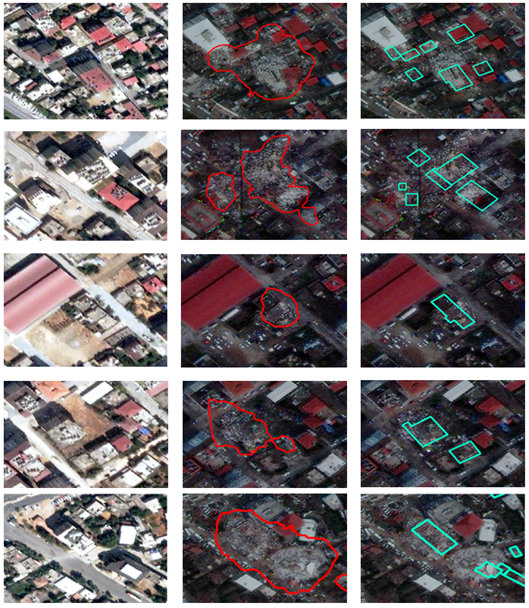
图5.倒塌房屋检测示例(Nurdagi)
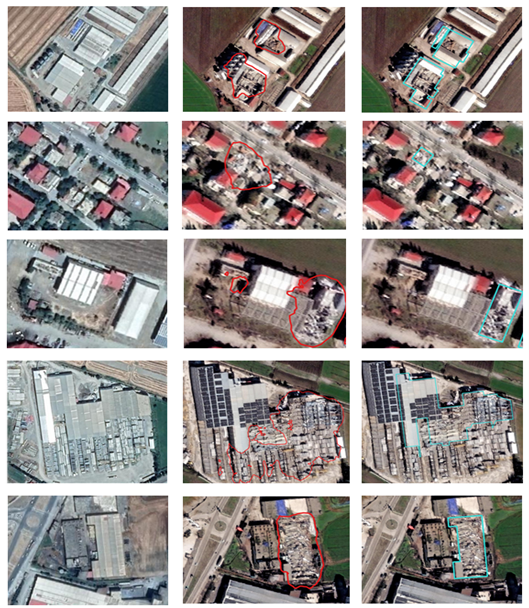
图6.倒塌房屋检测示例(Turkoglu)
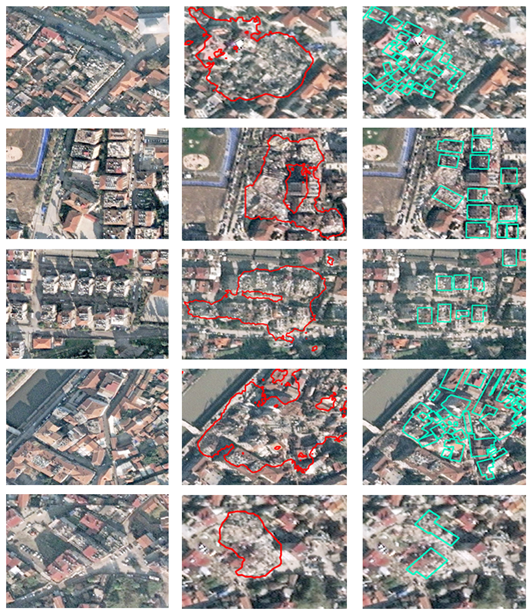
图7.倒塌房屋检测示例(Antakya)