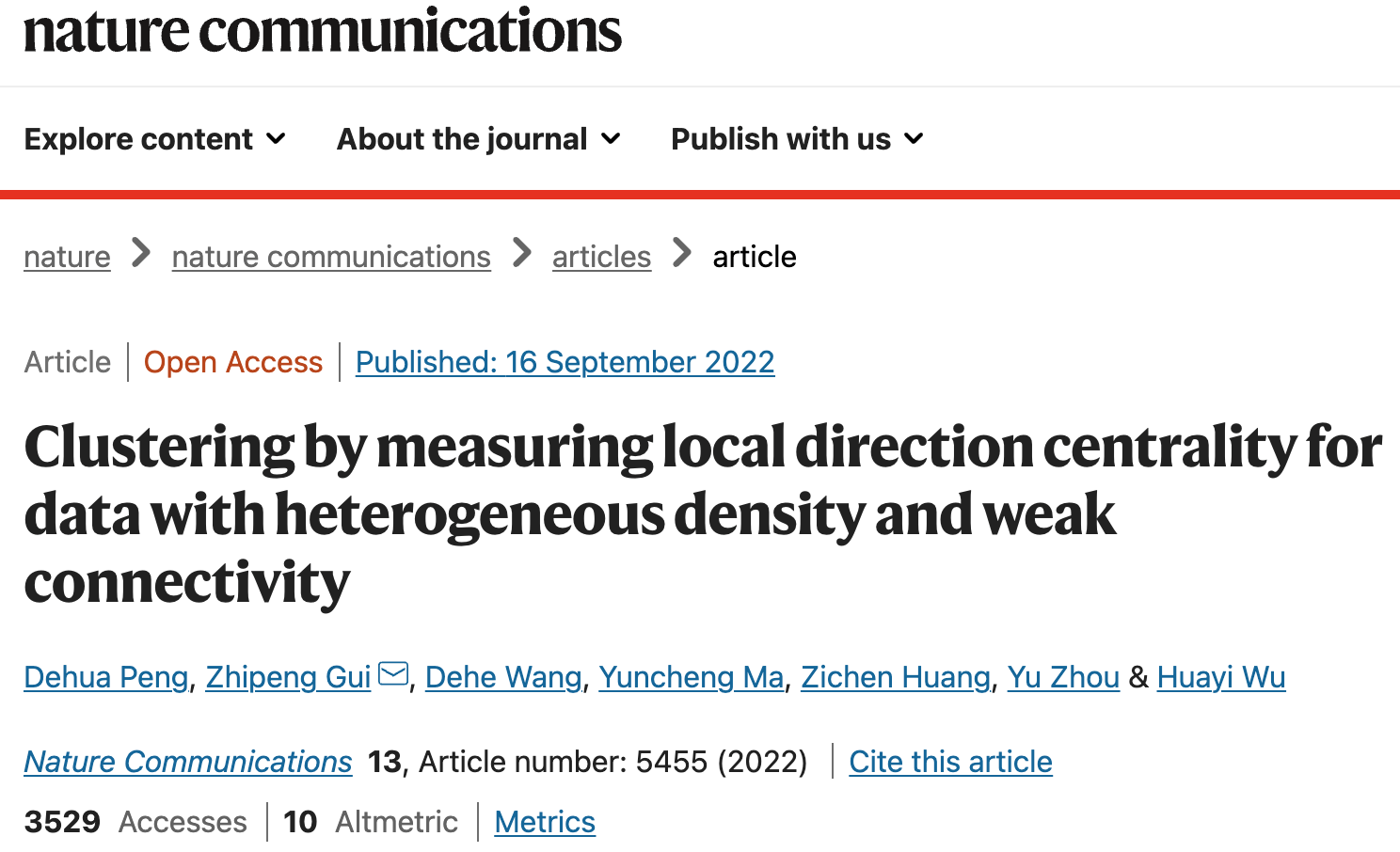
On September 16, Nature Communications published an article titled “Clustering by measuring local direction centrality for data with heterogeneous density and weak connectivity” of the research team of Associate Prof. Zhipeng Gui and Prof. Huayi Wu. This paper proposes a novel clustering algorithm CDC that can effectively overcome two common problems occur in clustering analysis, i.e., density heterogeneity and weak connectivity in data distribution, thereby improving the accuracy and stability of clustering. The effectiveness of the proposed algorithm is verified by comparing with the state-of-the-art benchmarks on various data types. An anonymous reviewer spoke highly of this work, and gave the positive comments that “The algorithm is extremely elegant, simple, and innovative…”.
The first author is Dehua Peng, who received bachelor’s degree from our school in Year 2018 and then enrolled as a master student, and now is a Ph.D. student in State Key Laboratory of Information Engineering in Surveying, Mapping and Remote Sensing (LIESMARS). Prof. Huayi Wu and Associate Prof. Zhipeng Gui are Mr. Peng’s first and second advisor respectively. Associate Prof. Zhipeng Gui is the corresponding author of this paper, he is now working in the department of Geographical Information Engineering of our school. The experimental analysis of cell type identification in this paper obtained substantial helps of Prof. Yu Zhou and Dr. Dehe Wang from the School of Life Sciences in our university. Part of computation in this work was done upon the computing resources in the Supercomputing Center of Wuhan University. Other co-authors include Ph.D. student Yuncheng Ma from LIESMARS, who just received his master degree from our school in this year, as well as master student Zichen Huang from our school. Meanwhile, this paper is supported by National Natural Science Foundation of China, National Key R&D Program of China and Zhizhuo Research Fund on Spatial-Temporal Artificial Intelligence.
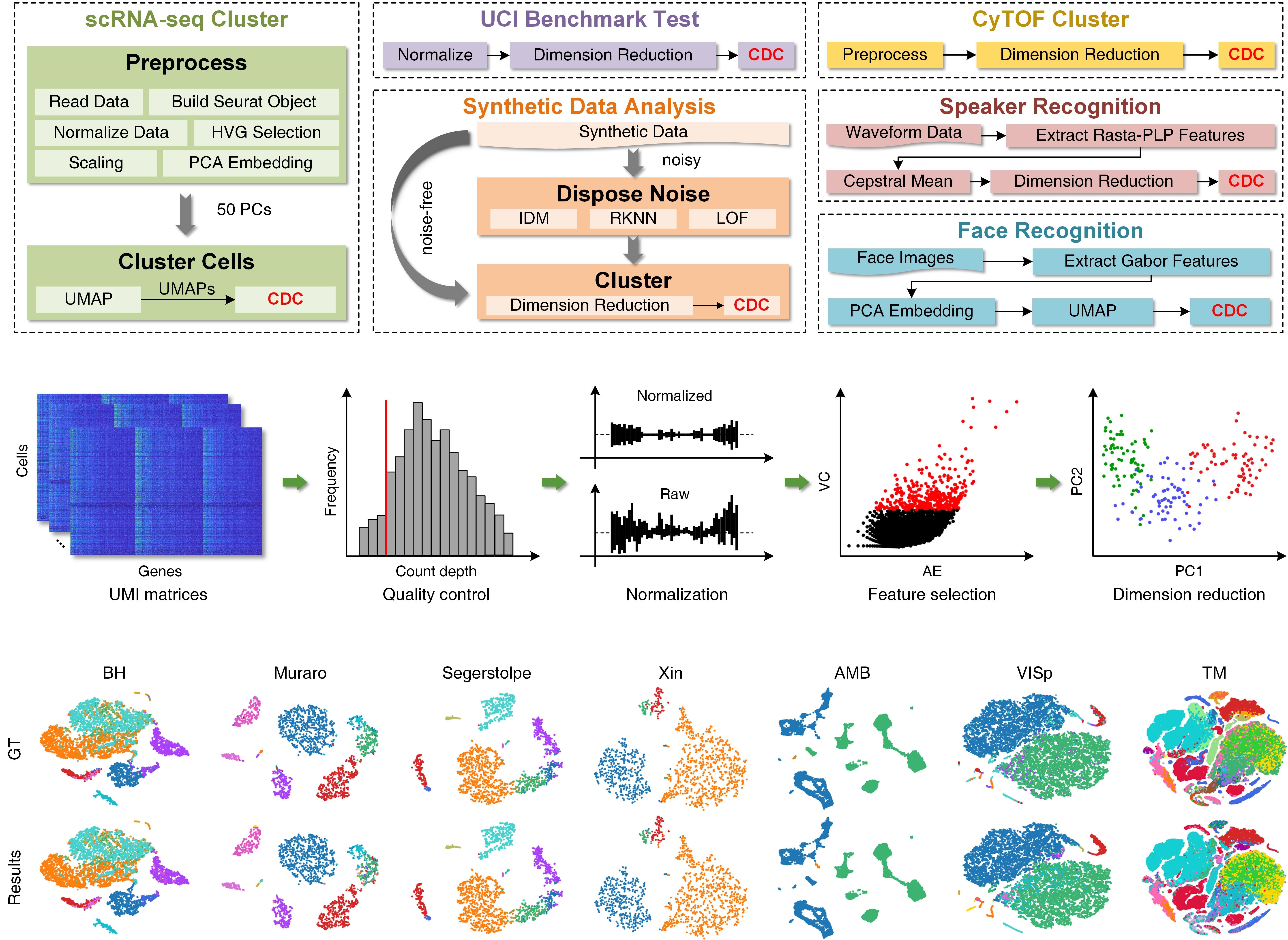
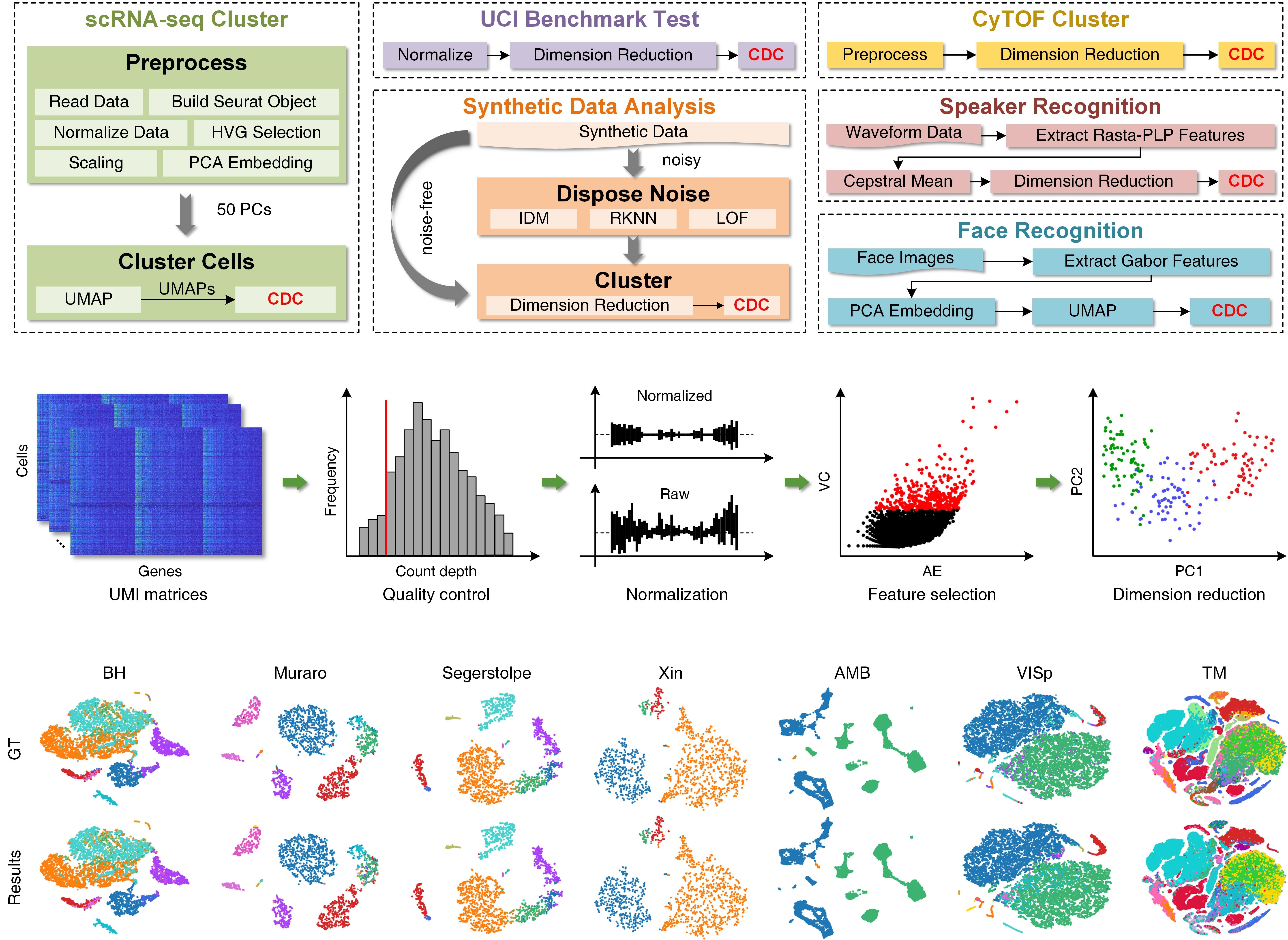
Figure 1. Applications of CDC, and the pipeline and clustering results on scRNA-seq datasets
Clustering is a powerful machine learning method for discovering similar patterns according to the proximity of elements in feature space. It is widely used in computer science, bioscience, geoscience, and social sciences. Although the state-of-the-art partition-based and connectivity-based clustering methods have been developed, weak connectivity and heterogeneous density in data impede their effectiveness. In this work, a novel boundary-seeking Clustering algorithm using the local Direction Centrality (CDC) is proposed. It adopts a density-independent metric based on the distribution of K-nearest neighbors (KNNs) to distinguish between internal and boundary points. The boundary points generate enclosed cages to bind the connections of internal points, thereby preventing cross-cluster connections and separating weakly-connected clusters. To validate the effectiveness, in this paper, CDC was compared with totally 38 specialized and versatile baselines on 47 datasets derived from different fields, including 15 scRNA-seq, two CyTOF, two speaker corpuses, eight UCI, one handwritten image, one face image and 17 synthetic datasets. Results demonstrated that CDC attains superior clustering accuracy and robust outcomes in a time efficient manner, and presented its great potentials in various applications.


Figure 2. Group photo of Dr. Zhipeng Gui’s research team during winter recreation
Paper Link:
https://www.nature.com/articles/s41467-022-33136-9